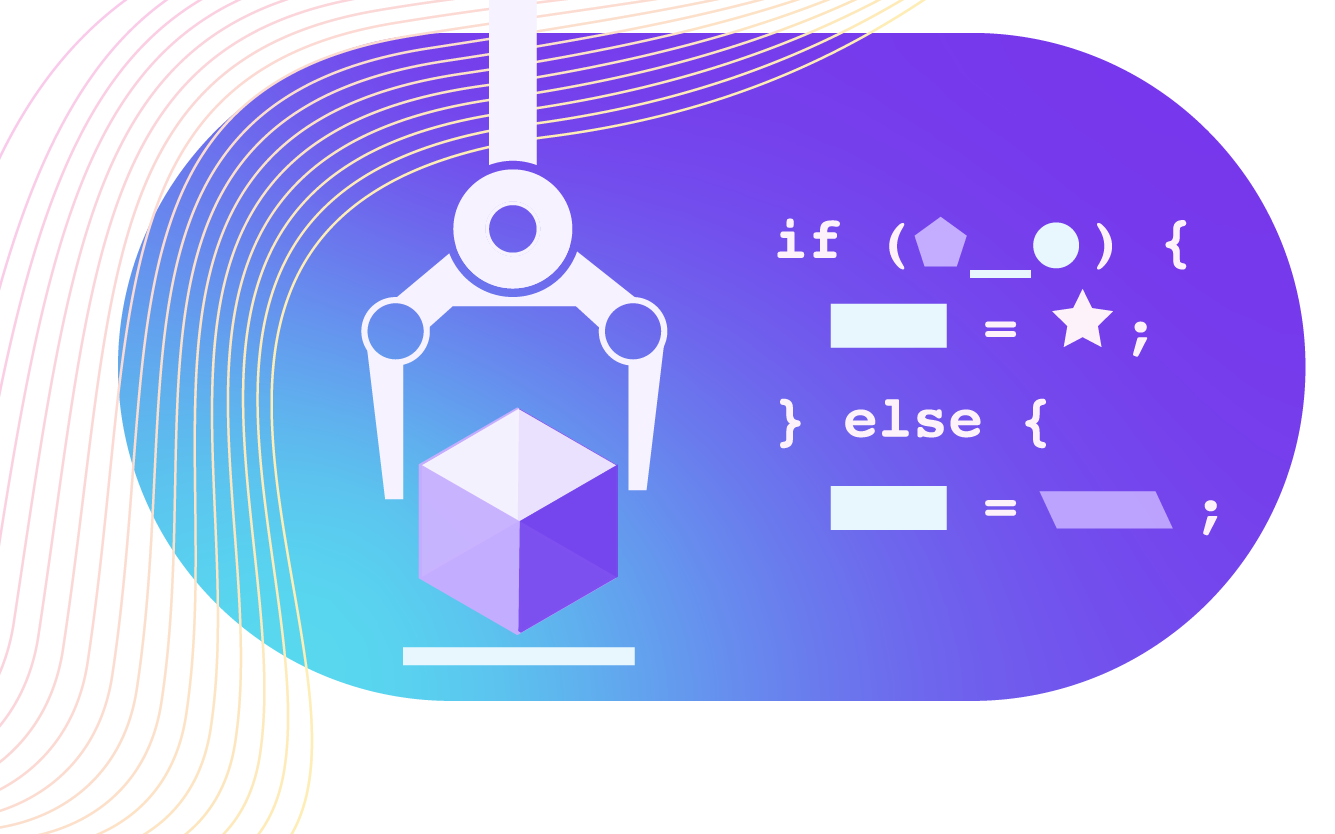
Why Proper Data Sourcing is Critical for Business Scalability
November 18, 2021
Table Of Contents
Many of today’s greatest innovations have been achieved through extrapolation and organization of data. Without hyper-analyzed data, we wouldn’t have the internet, smart phones, machine learning, predictive modeling, and much more. However, data sourcing remains the single most important step for data-based businesses and operations.
In this article, we will cover what proper data sourcing entails, why it matters, what can go wrong when data is not sourced correctly, and the steps we think are most important for every business to ensure data integrity.
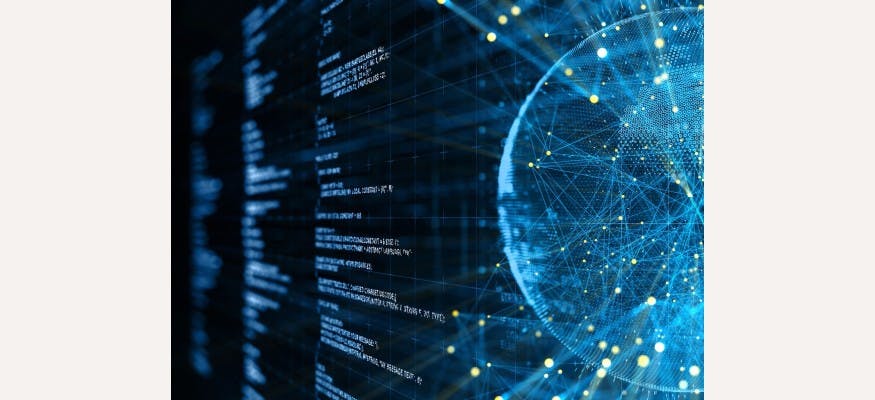
Why Properly-Sourced Data Matters
Properly-sourced data entails high levels of accuracy, reliability of communication and utilization, and compliance with data regulations. Some data operations today follow a quantity over quality approach. However, for use cases such as risk mitigation and fraud prevention, agencies need to validate identities to proactively identify bad actors and expedite valid requests. Therefore, even seemingly meaningless data points (including duplicates) must be digested.
When obtaining data, you also want high coverage - as measured by number of records - to ensure your information correctly reflects reality, and accurate linkages (astute connections to missing fragments) to ensure important information is not overlooked. Achieving high coverage with valuable linkages is often what sets apart top competitors in their respective fields.
Source Data vs Raw Data
Before we dive into distinctions, let’s make something clear: source data is not raw data. Rather, source data is a complete, single point of truth for a person, company, or entity that includes all compliantly-available information. Raw data can be very incomplete, but still correct (i.e. knowledge of only a person’s first name and their email). Many businesses today face the challenge of having a lot of leads in “raw” form, but their information on each person, company, or entity is extremely sparse or outdated.
Businesses that utilize strong source data with high coverage and intelligent linkages can easily enrich their raw data to have a single source of truth of source data for their operations.
For example, a marketing consultancy focused on public affairs and candidate communications struggled with gathering and consolidating enough information to identify activists, political leaders, and other influencers across platforms whose networks overlap with their clients’ target audience. It was increasingly challenging to communicate with hard-to-reach communities who might be missed by traditional outreach tactics like direct mail and robocalling.
Using a series of enrichment and person search APIs, they first enriched their current dataset to expand to all known, compliantly obtained data on the people already within their network. Then, they used a person search API to centralize their searches, eliminating the need for multiple data sources to craft full influencer profiles.
The result was expanded reach for client messages and a significant reduction of wasted time and effort, both of which are critical to time and cost-sensitive public affairs campaigns. These improved results yielded better outcomes for their public affairs clients, who were able to utilize a larger number of more effective influencers, and thereby distribute their messages more effectively to hard-to-reach communities.
For more information on this data journey, click here.
Properly sourced data saves businesses and people time, while increasing data-centric productivity across the board. When data is curated, presented, and exchanged in a format that is easily consumable with sufficient unique features, this allows businesses to to perform in their optimal manner. Every company needs to become a data company in some way, if they are not already, to thrive in today’s world and beyond.
What Goes Wrong When Data is Improperly Sourced
Silent Failure
Incorrect data is a silent failure. There aren’t alarms set to go off at a sign of a bad data record unless you conduct a semi-thorough analysis, such as cross-referencing your data against other information, or in some cases, doing inferences on your data set.
This is why data failures are usually noticed after the fact, when the problem is massive and PR is down the drain. In other words, these silent failures eventually turn into very loud problems. If you are running into issues within your company that you can’t quite nail down, it’s a good practice to follow your data, or even start over with the correct data.
Estimating the veracity of an individual piece of information in a dataset is very difficult and time consuming to do. This is often why data-centric companies rely on data sourcing or DaaS companies to ensure their data is bona fide and actionable.
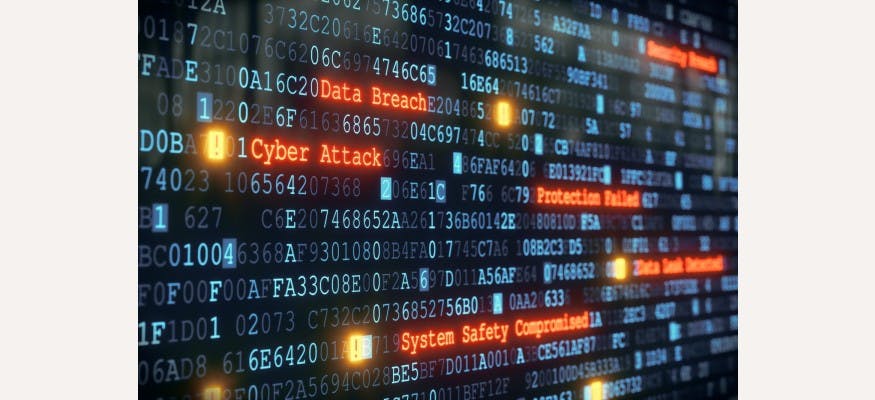
Bad Data = Bad Results
Every company is becoming or has already become data centric. This transition is critical to survival for almost nearly every industry. When data is incorrectly sourced or not taken seriously, you are welcoming false information into your business. At PDL, we refer to some of these records as “frankenstein records”.
Negligence of your source data reduces the trustworthiness of your data set and its reliability, and thus your trustworthiness in the eyes of your customers. Seemingly non data-centric business operations such as recruiting, product, and customer success are now heavily using data to improve their effectiveness.
The phrase “you are what you eat” applies here; the quality of your data now directly impacts your business’ bottom line. For example, companies that use datasets with high coverage (Google being a very apparent example) enable themselves to utilize their original information in innovative ways.
Propagation of False Information
There are cascading effects when data is incorrectly sourced. We have now seen the societal effects of misinformation when distributed through digital media. A sense of truth is critical now more than ever. Data sourcing involves a high degree of required responsibility for those that source the data and those that utilize it. Luckily, major moves have been made towards data security and compliance in recent years, but this field is ever-changing.
Incorrectly sourced or stored data, such as data sourced through non-vetted data providers, is not compliant or secure. Data files can potentially carry malware or malicious intent. Negligence in your data exchange can not only potentially hurt your business; it diminishes the quality of global security. When you obtain data for your business, pay special attention to where and how you are sourcing and storing the data, access keys, or passwords.
Data Sourcing Done Right
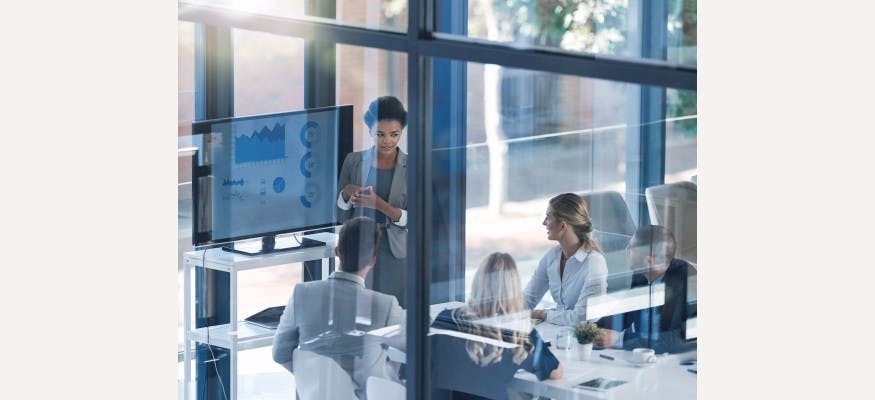
At PDL, we’ve found that curating accurate data is an ever-changing process. There is no single set of steps that can guarantee 100% confidence in data accuracy. In fact, Gartner reports that globally every month around 3% of data decays (36% annually). Data sourcers and curators need to be adaptive to trends and nuances in business and people data to stay ahead. DaaS companies today like People Data Labs are committed to this mission.
However, we can identify 5 vital best practices for sourcing data while maintaining its integrity:
Integrate quality checks into every single step of the data build process.
if profiles are merged, there need to be checks in place to estimate how well the merge went. While sometimes these checks seem tedious, they are necessary as 20% inaccuracy in one step could cause a 40% inaccuracy in the next inference. Quality checks also increase the awareness of the data builder to other relations and trends in the dataset.
Be conservative when estimating your data accuracy.
As discussed, data failures are silent. Because they are silent, they are hard to detect, and therefore, hard to reverse, especially when dealing with potentially millions of records at a time.
Set a high bar for the data you use. Make it prove to you that it is correct and accurate rather than assuming it is.
A common mistake made when analyzing data accuracy is running simple spot checks on datasets, then extrapolating over their entirety without ensuring there are no incorrect records in other parts. In other words, companies often fail by only checking a few records and assuming everything else also looks good. This causes a silent failure which then leads to propagation of false information.
To avoid this, ensure you are using both thorough spot checks and complimentary aggregate tests over the entire dataset together. For example, if you are verifying email, a spot check could be conducted to ensure emails do not bounce and they are current, while an aggregate test can be run to ensure a certain percentage match of the names.
Review sources diligently and enforce compliance.
When integrating from a new source, such as compliant public datasets and databases, do as much due diligence as possible. Understand who the provider is, where they got the data from, and if/how they manipulated the data in any way. The better your understanding, the better you will be able to judge its accuracy and reliability, which enables you to prepare accordingly.
Also note, if you store the data on prem, you are fully responsible for its security. Rules and regulations must be followed in the entirety of the data market, from sourcers to buyers. Ensure measures are taken to educate your data network and enforce compliance. Pay attention to licensing and rules on compliance for your use cases in your country and the world.
Apply simplifications where possible.
Formatting is everything when it comes to toggling large data sets. Apply blanket rules to your data, such as standardization and canonicalization, to enable fast queries, ease of visualization, and innovative adaptations.
To learn more about this and how People Data Labs sources data, read our blog, How People Data Labs Sources Data.
Remember that data is always changing.
Data, especially related to information about people, is dynamic and ever changing. This means that your data processes should always be evolving. If you’re not moving forwards, you’re probably moving backwards.
Companies that adopt these core strategies can exponentially improve efficiencies and strategize based on fact.
You’re Not Alone in Your Data Strategy
One of the world’s largest recruiting platforms struggled with enriching their candidate profiles that they provide for their clients while trying to simultaneously scale. With things changing so rapidly due workplace transitions spawned by the pandemic, the process of collecting, aggregating, matching and deduplicating their data became too large for their 14-developer strong team.
They enlisted a data partner that enabled them to focus on their offering rather than waste time finding a solution to their ever-evolving candidate data. With remote work and changes in people’s living situations quickly on the rise, workable enriched their existing people data to be more accurate, thus increasing the overall quality of their matching capabilities. This also enabled them to find new, obscure candidates by utilizing similar datasets to surface net-new candidates.
For more information on this data journey, click here.
If you’re orchestrating a data-centric business, keep in mind you’re not alone in this effort. Speak to one of our data consultants to form an understanding of how your business can put data to use, or try our API for free today.
Like what you read? Scroll down and subscribe to our newsletter to receive monthly updates with our latest content.
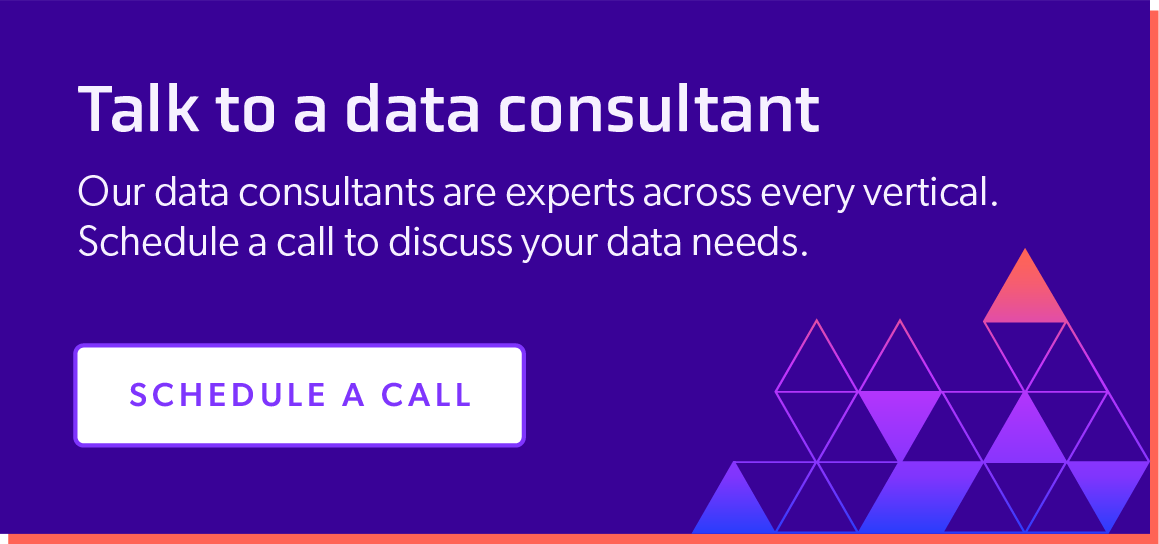