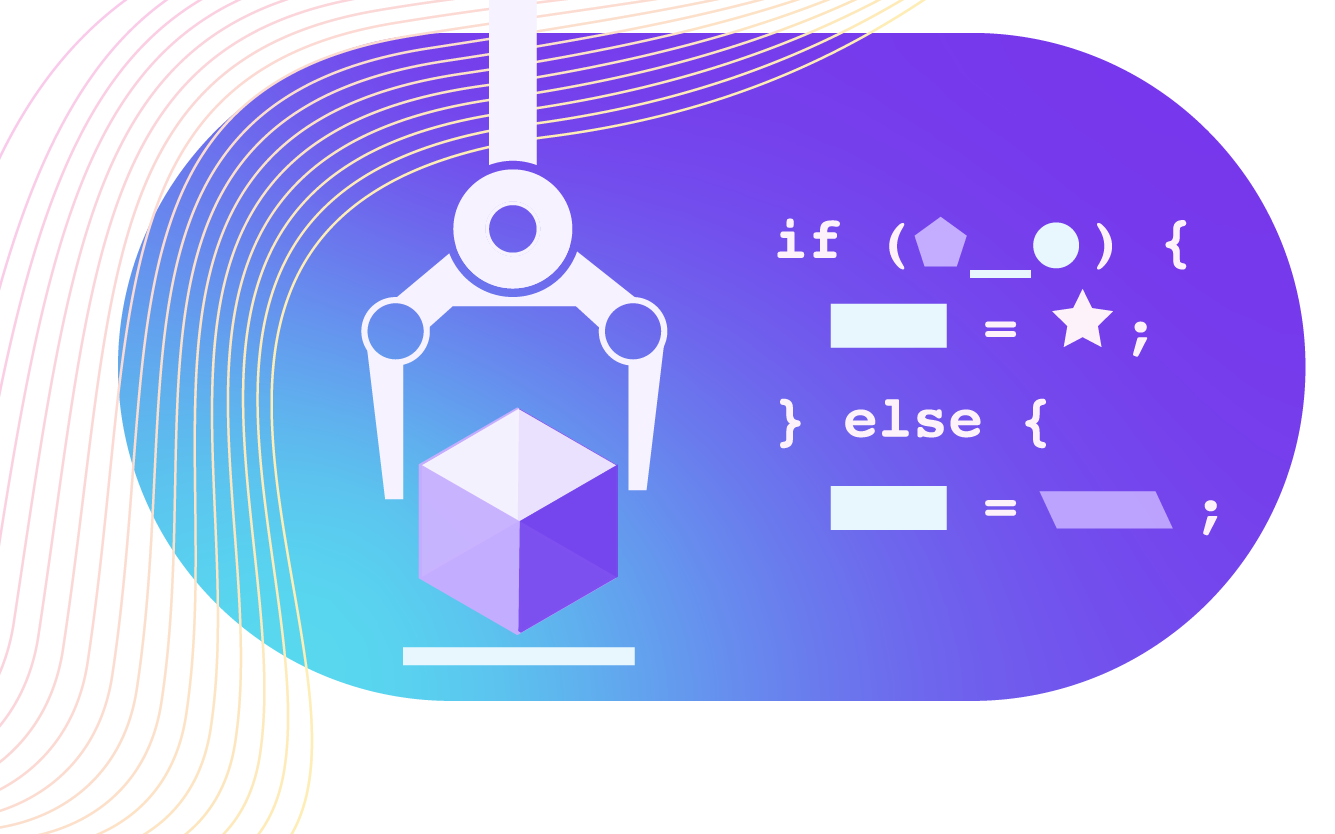
Where Does Data Get its Value?
August 18, 2021
Table Of Contents
In the early information age, the approach to data was quantity over quality; obtaining as much data as you could with the assumption that someone or something would analyze it after the fact. Now, well into the 21st century, we know that data volume isn’t everything. In fact, out-of-date data with little to no context can actually decrease the efficiency of your processes and the accuracy of your insights. This realization changes the way we assign value to data.
How to Value Data
Today’s data should be valued not by volume, but by our ability to take action on it. In industry and connectivity spaces, edge computing enables faster processing and relaying of information (data). For developers, automating analysis of immediate data and orchestrating collaboration with humans proves the value of the software. And, for advertising agencies, knowledge of prospects’ online behavior greatly improves outreach effectiveness.
At PDL, we grade data quality based on the number of linkages – how many data points we can confidently link together – for each person or company record. In simple terms, records that contain more information via correct linkages are more valuable. For example, the name Franklin D. Roosevelt means nothing alone, but knowledge that he was the 32nd president of the United States is an essential linkage that contains real value, perhaps at your next trivia contest.
However, only correct linkages create value. Even one incorrect linkage can cause failure. For example, customers might need email addresses based on name and location, but if a data set has incorrect linkage parameters (mismatched names and addresses), they will find the wrong records and miss the right ones.
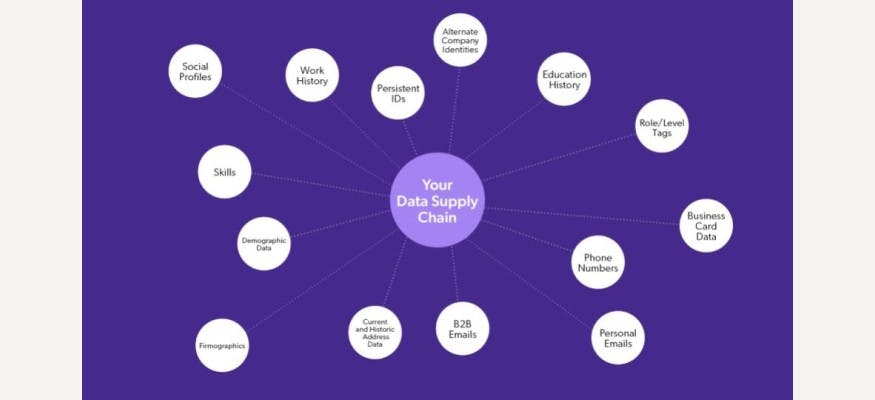
Data Dwarves
An easier way to understand the relationship between data and the companies that source it is to apply the analogy of dwarves. In fantasy and lore, dwarves are known for mining, smithing, accumulation, protection, and selling of valuable minerals like gold, silver, other precious metals and ore. Just like these minerals, data has inherent value, but when you process it and apply linkages (combine minerals/process in smithy), the result can be an alloy of even greater value.
In our data-driven world, we rely on data companies to mine, build upon, preserve, update, and facilitate the exchange of valuable data. If this process isn’t thorough and continuous, the banks and libraries of value and knowledge are lost to the orks and dragons.
The Risk of Inferences
Data is often compared to commodities, like oil and gold, because of the mistaken perception that one can “strike it rich” if they uncover a sufficient supply. But, there’s a reason that droves of people aren’t hitching up their proverbial covered wagons and leaving their jobs to make their fortune in whatever location is the data-world equivalent of the black hills or the oil fields of Texas: data is complex, expensive to process, and challenging to build.
To apply linkages to data, a deduction, known as an inference, must be made about how to connect the data points. However, each inference incurs a risk. Some inferences have an extremely low risk, (such as matching a location to a person’s name within a single zip code) but the further down the rabbit hole you go, the more exponentially complex it gets. The stakes are high, as a flawed linkage can have cascading effects in applications or operations in the real world.
Data providers who are lax in creating these linkages run the risk of producing flawed or incoherent records. We call these “Frankenstein records.” They might resemble a person, but like Mary Shelley’s literary monster, they contain elements of multiple individuals. When data on multiple people is tied together in this fashion, it can render that record unusable or even application-breaking.
It’s important to note that the less constrained an inference is, the more susceptible it is to incorrect data. For example, inferring location based on a zip code is low risk, but inferring a job title based on a zip code is high risk, with many more complicated variables to account for. Also, an inference should not be confused with a simple educated guess. There are more specific and referenceable deductions that go into a proper inference.
In our case, given the volume of data PDL takes in, we often have many raw records with 3-4 profiles of disparate information that relates to the same person. While we could link these together based on name, for example, this would create many false positive linkages. In these cases, we take care to present data as authentically as possible with the minimal amount of modification.
Proper Discretion When Conducting Inferences
If inferences are so risky, why make them at all? In contrast, when data providers make inferences, why not just combine as much information as possible? No one likes duplicates. Right?
Wrong.
In certain situations, such as fraud detection, agencies may find great value in duplicate or abnormal data. For example, a credit card agency might be interested in knowing every possible location associated with a person, so that they can flag suspicious behavior to their users (such as a large purchase for a boat in a location the user has never lived). Or, for a less dire example, if a new record appears that has the same information except for a different verified email, it could be inferred that that person has created a new email, and the old email could be categorized as historical data. We will cover more use cases of historical data in upcoming blogs.
Inferences possess the ability to make or break everyday operations for any team or application that interfaces with data (pretty much everything nowadays). It is the responsibility of those who are interfacing with sensitive and valuable data to make correct inferences to ensure that the truth is not diluted.
Onward
If your business relies on data, as most now do, we recommend not going it alone. There are thousands of tools and experts out there willing to help you along the way.
If you want to learn more and delve into the way we ‘mine’ and ‘smith’ our data, speak to one of our experts today!
Like what you read? Scroll down and subscribe to our newsletter to receive monthly updates with our latest content.
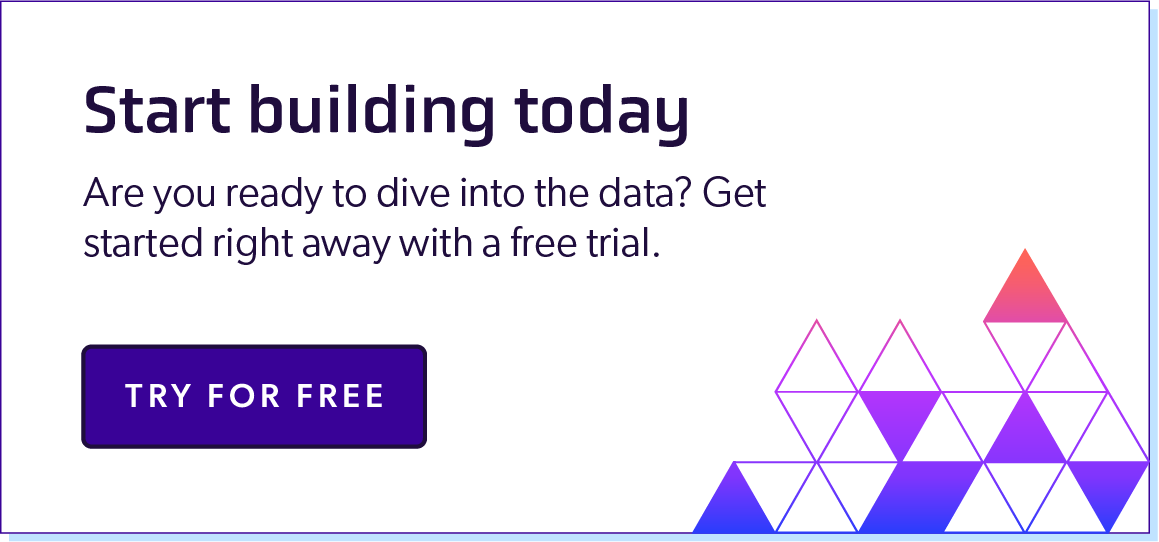