How to Build a Custom Audience with PDL
July 15, 2022
Table Of Contents
Before You Start
Goal: Build a Custom Audience list using Python and the PDL Company Enrichment & Person Search APIs.
Level: Advanced – You know how to make API calls in Python and parse the response.
What You'll Need:
To run the code samples in this tutorial, install Python 3.6+, requests, and pandas
Credit Usage: If you run the code as-is in this tutorial, it will use:
25 Company Enrichment Credits
50 Person Search Credits
Scenario
In this tutorial, we will walk through how to use PDL’s Company Enrichment and Person Search APIs to build a Custom Audience of decision makers at Software as a Service (SaaS) companies based in New York City.
What is a Custom Audience?
In marketing campaigns, it can be helpful to create Custom Audiences - lists of people who share certain characteristics that mean they may be interested in your product. Custom Audiences are frequently used in social media marketing campaigns (ex: Facebook and Twitter), since they can send tailored material directly to the people most likely to buy your product or service.
Step 1: Enrich Company Data from Initial List
Let’s assume that we were given a CSV file of potential NYC-based tech companies to build our Custom Audience from. However, all we have to go off of is very basic information for each company.
Since our Custom Audience requirements are more specific than the information we were given, this is an excellent opportunity to use PDL's Company Enrichment API.
Let’s pull PDL’s records for each company using the following code:
def enrich_company(name: str, website: str, linkedin: str) -> dict:
resp = requests.get(
'https://api.peopledatalabs.com/v5/company/enrich',
headers=headers,
params={
'name': name,
'website': website,
'profile': linkedin
}
)
if resp.status_code != 200:
raise ValueError(f'Request to {resp.request.url} failed. '
f'Response: {resp.status_code} - {resp.text}')
return resp.json()
Call the function like this:
# convert the CSV to a pandas DataFrame
initial_df = pd.read_csv(initial_list, header=0)
# call the Company Enrichment API for each row in the DataFrame
# and save the responses as a new DataFrame
enriched_df = initial_df.apply(
lambda row: enrich_company(
row['name'],
row['website'],
row['linkedin_url']
),
result_type='expand',
axis=1
)
print(enriched_df.head())
print(f'Enriched Data Size: {enriched_df.shape}')
Once you run the code, you should see:
status ... likelihood
0 200 ... 10
1 200 ... 10
2 200 ... 10
3 200 ... 10
4 200 ... 10
[5 rows x 26 columns]
Enriched Data Size: (25, 26)
Step 2: Filter Company List using Criteria
The Company Enrichment API response contains a lot of information about each company in a standardized format. For more information on all the data in the Company Enrichment API response, see Company Schema.
From our Scenario, we know companies in our Custom Audience should be:
Based in New York City
In the Software as a Service (SaaS) industry
Since our initial list already provided only NYC-based companies, we can skip filtering for that and just determine which companies work in SaaS.
PDL's tags field is a great way to find a company’s major associations, so let’s use it.
def filter_for_tag(to_filter: pd.DataFrame, tags: [str]) -> pd.DataFrame:
# create a mask (True/False) for if each row has any of the tags
mask = to_filter['tags'].dropna().apply(
lambda row: any([tag in row for tag in tags]))
# apply mask to filter DataFrame
return to_filter[mask]
Call the function on the enriched DataFrame:
saas_df = filter_for_tag(enriched_df, ['saas'])
print(saas_df.head())
print(f'Found {len(saas_df.index)} companies with "saas" tag')
Now we've filtered our initial list down to three NYC SaaS companies.
status name ... affiliated_profiles likelihood
8 200 pipedrive ... [] 10
10 200 datadog ... ['openview-'] 10
22 200 invisionapp inc ... [] 10
[3 rows x 27 columns]
Found 3 companies with "saas" tag
Step 3: Find Decision Makers at Each Company
Now that we have our list, we want to find decision makers at the companies to advertise to. In this tutorial we define “decision makers” as all employees whose job title level is Director, VP, Partner, Owner, or “CXO” (CEO, CFO, CTO, etc.).
Let’s use PDL’s Person Search API to search each company for all employees that match our criteria. Each employee should:
Work for one of our targeted companies
Be a decision maker
Have a known work email (so our marketing material can reach them)
The Person Search API supports queries written in SQL or Elasticsearch. PDL strongly recommends using Elasticsearch, since it is what our architecture is based on and supports more detailed queries.
For more information on writing queries using Elasticsearch, see https://blog.peopledatalabs.com/post/query-builder-tutorial.
In Elasticsearch, the query for our custom audience looks like this:
search_query = {
'query': {
'bool': {
'must': [
# work for one of our targeted companies
{'terms': {'job_company_linkedin_url': company_linkedins}},
# be a decision maker
{'terms': {'job_title_levels': ['owner', 'director', 'cxo', 'vp', 'partner']}},
# have a known work email
{'exists': {'field': 'work_email'}}
]
}
}
}
We can send the request to the Person Search API like this:
⚠️ Warning: High API Credit Usage ⚠️
This code snippet finds 50 profiles, costing 50 Person Search credits. If your plan’s rate limit is less than that, you can change the size
parameter in the request to limit the number of responses or contact support@peopledatalabs.com to increase your limit.
def get_decision_makers(company_linkedins: [str]) -> pd.DataFrame:
# get 50 matches
resp = requests.post(
'https://api.peopledatalabs.com/v5/person/search',
headers=headers,
json={
'query': search_query,
'size': 50, # number between 1-100
'dataset': 'resume'
}
)
if resp.status_code != 200:
raise ValueError(f'Request to {resp.request.url} failed. '
f'Response: {resp.status_code} - {resp.text}')
return pd.DataFrame(resp.json()['data'])
Call the function like this:
companies = saas_df['linkedin_url'].values.tolist()
audience_df = get_decision_makers(companies)
print(f'Audience Data Size: {audience_df.shape}')
print(f'Found {len(audience_df.index)} people for Custom Audience')
Which gives us 50 PDL profiles of decision makers at our target companies as a pandas DataFrame:
Audience Data Size: (50, 73)
Found 50 people for Custom Audience
We’ve found our Custom Audience!
Step 4: Export the Custom Audience List
Depending on the marketing tool we want to use, we will export our Custom Audience DataFrame according to the tool's requirements.
For example, Twitter's Custom Audiences Tool supports a CSV of emails that we can build by exporting the DataFrame like this:
audience_df['work_email'].to_csv(filename, header=None, index=False)
Similarly, if we wanted to use Facebook's Custom Audiences Tool, we can build a CSV according to their specs like so:
headers = ['email', 'phone', 'fn', 'ln']
fields = ['work_email', 'mobile_phone', 'first_name', 'last_name']
audience_df[fields].to_csv(filename, header=headers, index=False)
What We’ve Accomplished
In this tutorial, we took a basic list of potential target companies and used it to build a highly targeted Custom Audience that we can use for an advertising campaign.
We walked through how to:
Run a starting list of companies through the PDL Company Enrichment API to get more information about them
Filter the dataset to target just the companies tagged “SaaS”
Use the PDL Person Search API to find decision makers working at each target company
Turn the person matches into Custom Audience CSVs that can be uploaded to Twitter and Facebook
See the full code for this tutorial here: custom_audience_generator.py
For More Information:
Questions? Reach out to support@peopledatalabs.com, we’ll be happy to help!
Noticed any issues with this tutorial? Let us know at support@peopledatalabs.com!
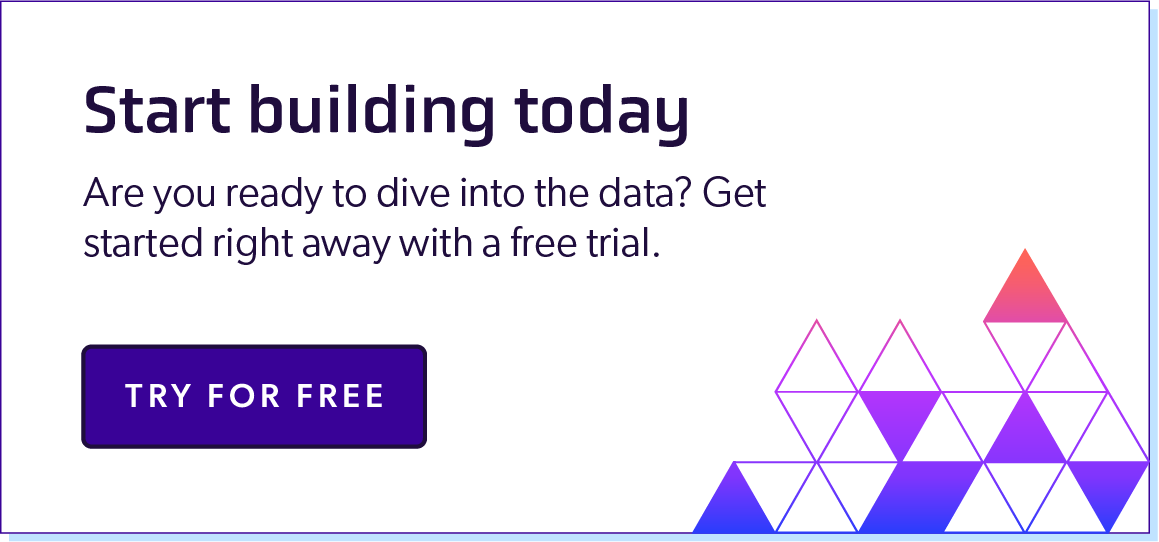